When robots take over the work: from transporting to kicking
Researchers and companies in Adlershof are hands-on involved in the development
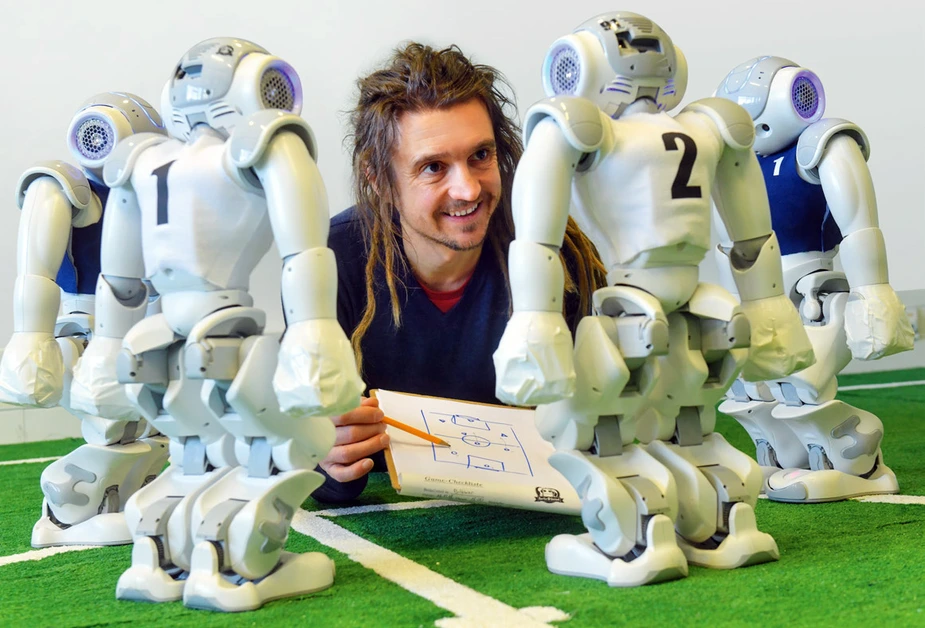
A tense hush descends as the Berlin United team scuffles in front of the opposing goal. Will the player make the shot and bring the game to a draw? He shoots, he scores and the crowd roars wildly: Draaaw!
They could still work on their passing game. After the final whistle, the football trainers Pál Dárdai and Urs Fischer are the first to admit it. The “coach” of Team United, Heinrich Mellmann, is also well aware that cooperation is still one of the crucial issues his team has to work on. Although, his team is in a whole different league from the Hertha BSC or the 1st FC Union Berlin: they are the five half-metre-tall humanoid robots of the Humboldt-Universität zu Berlin (HU) competing in the RoboCup. Here, international research teams compete with all kinds of robot classes in football.
“With the RoboCup, we want to promote the development of intelligent robots,” explains the computer scientist and mathematician Mellmann, who earned his doctorate in the “Adaptive Systems” group of Professor Verena Hafner. “Football is a perfect problem on which to develop new ways for robots to find their way around a real environment on their own.”
Over the course of years, the researchers have continually increased the complexity of the environment. The ball is now black and white instead of red; the goals are white instead of brightly coloured; and they play on artificial grass instead of carpet. The game is now played under natural lighting conditions. This is a real challenge for the limited sensors – the NAO robots are equipped with only one camera for spatial orientation – and this challenge has to be overcome by clever programming.
The robots move across the field in small, toddling steps. Each is controlled by its own computer inside the head. When a player falls down, it gets straight back up with skilful movements. Where is the ball? Where is the goal? Where are the other players? How do you have to kick the ball to send it the right way?
What humans can already do almost intuitively, the robots will one day also be able to do “without separation of mind and body,” as Mellmann puts it. This will be accomplished using methods of artificial intelligence (AI), mainly and more specifically: machine learning. Much how professional footballers have to practice their game structures, passes and goal shots, the NAOs also improve themselves through gaining experience.
“Behind AI is a completely different kind of programming, which we are currently developing and researching,” says Professor Holger Schlingloff of the HU and chief scientist of the System Quality Center at the Fraunhofer FOKUS. This is where, for example, the method known as Deep Learning comes in. Characteristic of this method is that it does not analyse all possible options, rather it learns from many, many examples. No programmer ever defines what action has to be performed in what situation.
And yet, to gain a certain level of control over the quality of the computer-made decisions, the researchers are finding answers to questions such as: How does this learning work in the first place? How can we define a decent feedback method for the learning system? How do you prevent it from learning the wrong things?
One of Schlingloff’s specialty fields is safety. He is also contributing his knowledge to a joint research project with the company InSystems Automation. Software quality is an issue that drives Jan Stefan Zernickel as well. The computer scientist at InSystems develops transport robots. These busy helpers whizz through industrial production halls, transporting boxes and bringing semi-finished products from one production station to the next. Hence the name “proANT” (Automatic Navigating Transport robots – in honour of their industrious namesake, the ant). One day, they will replace conventional transporting technologies in factories.
“This system of individual robots is efficient in many respects: they save space, which is a precious commodity in factories as well. They are scalable and flexible in how they can be used, given that production will become increasingly individual – with lower part volumes and more frequent system reconfigurations,” Zernickel lists the benefits. They are also more flexible and robust than line-following or centrally controlled robots.
The robot columns will one day organise themselves, is the goal. That means, they will decide among themselves who does what job and when. Zernickel speaks of “cooperative” and “collaborative” behaviour.
Fitted with all kinds of sensors that deliver information about the operating status, provide orientation in the room and avoid collisions with people and machines, the robots can process their sensor data with their own on-board computer and communicate with each other via Wi-Fi. The only instructions they still need to be given are global objectives, for example: machine A needs x screws in twenty minutes. Then it is again their task to figure out: Who is closest? Who is already doing other tasks? Is there enough battery left?
“With the help of AI, we want to detect patterns in the operation – both in the collective behaviour and in the self-optimisation of each individual machine,” says Zernickel. For example, when it comes to fine positioning to pick up a box of materials, it is important to analyse all sensor data.
Despite the current euphoria surrounding AI and its possibilities – there are also risks: The robot researchers Mellmann and Zernickel stress that they are only just setting out in this direction. “You have to consider carefully when you would use AI. A large quantity of training data and good feedback are especially important,” Schlingloff adds. They do not believe that the technology can be stopped any more, in this age of ever larger volumes of available data. That makes it all the more important to include the public in the developments, Mellmann believes.
Byn Dr. Uta Deffke for Adlershof Journal